FAIRsFAIR aimed to supply practical solutions for the implementation of the FAIR data principles throughout the research data life cycle. Between March and November 2019, FAIRsFAIR ran an open consultation, and undertook desk research and a small number of interviews to assess the current FAIR policy and practice landscape with regard to:
- the range of policies that influence the way researchers work
- the range of initiatives to enable different levels of maturity with regard to FAIR practices across disciplines
- the sources of support currently available to researchers
Who took part in the open consultation?
A total of 106 responses were received. The majority of responses were received from:
- staff working in Universities (44%)
- Research Infrastructure staff (30%)
- Research Performing Organisations (10%) and ‘other’ (9%).
The majority of responses came from countries that currently demonstrate a relatively high engagement with Open Science and the FAIR principles including the UK (19%), Netherlands (13%), Germany (12%), Finland and France (9% each), Ireland, Italy, Spain and Sweden (5% each).
Policy related findings
Policies are a crucial component in the FAIR ecosystem. In addition to the open consultation, FAIRsFAIR carried out an analysis of 42 current data policies at various levels (national, funder, publisher, institutional) to identify policy elements that support or hinder FAIR data practice and to share examples of good practice. To support comparison across the data policies of different stakeholders, a standard set of policy characterisation features was developed to help us assess progress against the priority and supporting recommendations outlined in Turning FAIR into Reality. The results of the analysis are presented in D3.1 FAIR Policy Landscape Analysis which provides a snapshot of the policy landscape in November 2019. A selection of the main findings are provided below. A selection of the main findings are provided below.
♦ Policies are not currently structured for machine readability
To enable machine-actionable workflows to be carried out in a FAIR ecosystem, it will be crucial that the policies governing data sharing are comparable and can be interpreted unambiguously by both humans and machines. To this end, there is a need to agree on a set of common policy elements that will be consistently described using a structured markup schema.
♦ Some stakeholders’ policies are more influential on researchers’ behaviour than others
Based on the results of the open consultation, the policies of funding bodies are the most influential on researchers’ practices followed by those of publishers and journals. Community norms also have significant influence on researchers’ practices as do the policies of Government or National Ministries. Policies at the Research Performing Organisation (RPO) and Research Infrastructure level have slightly less influence over researchers’ practices and the least influential policies appear to be those of data repositories.
♦ Policies should be aligned with FAIR either explicitly or implicitly
While many of the funding body and publisher/journal data policies we reviewed contain FAIR-related elements, less than half specifically refer to the FAIR principles explicitly. One of the publishers interviewed stated that they do not specifically reference the FAIR principles even though their policy aligns with them because they feel the term is confusing for researchers. While explicit reference to FAIR may not be necessary in all policies, related guidance and support should make clear how the policy aligns with FAIR implicitly.
Practice related findings
Data Stewards play an integral role in enabling good research data management practice and help to realise the production FAIR data available for reuse. The majority of research performing organisations that participated in the open consultation do provide in-house research data management support to varying degrees. Some of the key findings are provided below.
♦ Timing of support provision is crucial
We must identify points over the full data lifecycle where RDM support is most effective for enabling FAIR data production. This is particularly true when it comes to selecting and implementing metadata standards to support interoperability and interdisciplinary reuse.
♦ The availability of local support has a positive influence on researcher behaviour
The open consultation showed that the availability of support to help researchers make their data FAIR is considered to be the most positive policy factor influencing researchers’ behaviour with more than 93% of respondents rating this factor as ‘very’ or ‘quite’ positive. Encouragingly, the open consultation also showed that more than two thirds of respondents’ organisations currently provide in-house support.
♦ Who provides support?
Based on the findings of the open consultation, RDM support for making data FAIR is most frequently provided centrally by Library or Research Support units in organisations. However many institutions are now introducing dedicated support at the faculty or departmental level. Organisations with limited resources can still provide an essential sign-posting service to a growing body of open training and learning resources such as those developed by Research Infrastructures and related Cluster projects (e.g., ELIXIR, SSHOC).
♦ Data Stewards tend to wear more than one hat!
Respondents identified with a variety of roles, 49% indicated that they fill several roles at their institutions. In many cases, respondents indicated that they held between three and five roles within their organisation. Of the 51% of respondents that have a unique role, the majority (14%) working in research support and liaison followed by policy makers or senior managers (11%) and data stewards or research data librarians (9%).
Landscape reports
Based on the initial landscaping activity, a number of reports have been published along with practical recommendations targeting policy makers, data stewards and repository managers.
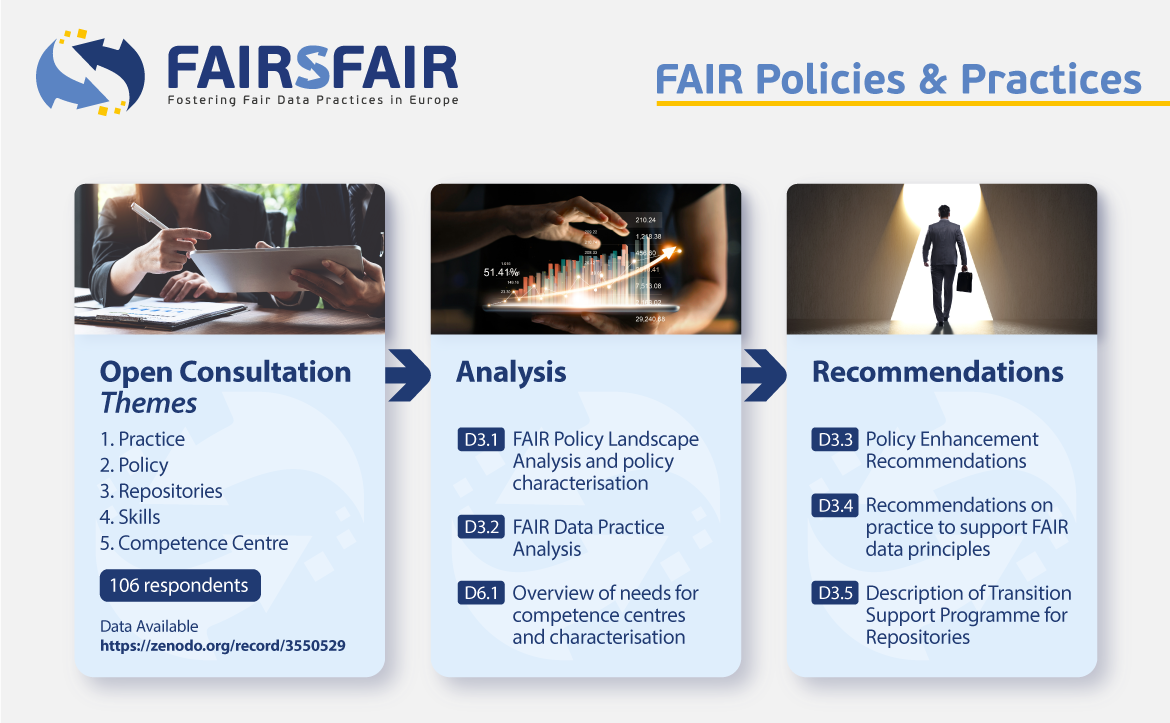
Relevant outputs
- FAIRsFAIR Policy and Practice Survey 2019 data for D3.1, D3.2 and D6.1. The survey questions of presented in the open consultation on Policy and Practice and the resulting data are available for reuse. The open consultation was part of our initial landscape review and ran from August 2 - September 27, 2019.
- FAIRsFAIR Policy Characterisation Data for D3.1. As part of our landscape assessment, we reviewed 42 policies from different stakeholders (funders, publishers, HEIs) that had a relatively high level of engagement with the FAIR principles to see how far they reflected the stakeholder-specific actions as recommended in Turning FAIR Into Reality. The resulting data are available for reuse.
- D3.1 FAIR Policy Landscape Analysis. This document provides a snapshot of the data policy landscape at various levels (national, funder, publisher, institutional) in 2019 and identifies policy elements that support or hinder FAIR data practice.
- D3.2 FAIR Data Practice Analysis. This document provides an analysis of practices to support FAIR data production within a broad selection of research disciplines and research data repositories.
- D3.3 Policy Enhancement Recommendations. Based on the outcomes of D3.1, FAIRsFAIR prepared a series of practical recommendations for policy enhancement to support the realisation of a FAIR ecosystem.
- D6.1 Overview of needs for competence centres and characterisation. This document explores competence centre requirements, provides an overview of current competnce centres available, and shares examples of good practice.
- D3.4 Recommendations on practice to support FAIR data principles. Building upon the findings outlined in D3.2, FAIRsFAIR developed a set of recommendations for practical actions to be used to inform the development of guidance resources to support further adoption of FAIR data standards and practices by research communities.
- D3.5 Description of FAIRsFAIR's Transition Support Programme for Repositories. This document describes the range of FAIRsFAIR support activities intended to help repositories on their journey towards better alignment with the FAIR data principles.
- D3.8 Final report on policy and practice recommendations and support. The report describes a range of WP3 support, resources and guidance developed to support our policy and practice recommendations. The report describes support for three key stakeholder groups - policy makers, research communities and repositories.